As the world of generative artificial intelligence (AI) continues to evolve, Amazon Web Services (AWS) is taking a distinct approach that sets it apart from competitors like Microsoft and Google. While Microsoft Azure and Google Cloud have focused on large-scale, one-size-fits-all models, AWS is concentrating on an AI ecosystem that combines proprietary large language models (LLMs) with third-party models to offer a more tailored and specialized experience for its customers.
Microsoft has centered its AI strategy around OpenAI and its popular GPT-n foundational models, with GPT-4 being utilized across its Copilot branded productivity tools. Google Cloud, on the other hand, has committed to providing a range of AI models to its customers, while also focusing on large-scale models like PaLM 2 to power its search chatbot Bard.
In contrast, AWS is emphasizing its commitment to providing customers with the widest range of AI tools, thus distancing itself from other hyperscale AI providers. The company’s strategy allows smaller firms to embrace generative AI without having to settle for “jack of all trades” models that lack specialization. Amazon’s Titan LLMs, for example, come pre-trained to filter out profanity and hate speech.
During the keynote presentation at the AWS Summit London conference, AWS showcased an example of a digital marketing firm using various AI models to execute a product campaign. The firm used Anthropic’s Claude AI assistant for product descriptions, StableDiffusion for generating product images, AI21’s Jurassic-1 LLM for social media copy, and AWS’ own Titan foundation model for SEO-optimized terms.
All of these models are accessible through Amazon Bedrock, an ecosystem of AI and machine learning accessible via APIs. This platform enables companies to pick and choose which data is passed to which model, resulting in tailored outputs. Swami Sivasubramanian, VP of Database, Analytics, and ML at AWS, emphasized that with Amazon SageMaker JumpStart, customers can either embrace pre-trained models or train their own ML tools using AWS’s cloud architecture.
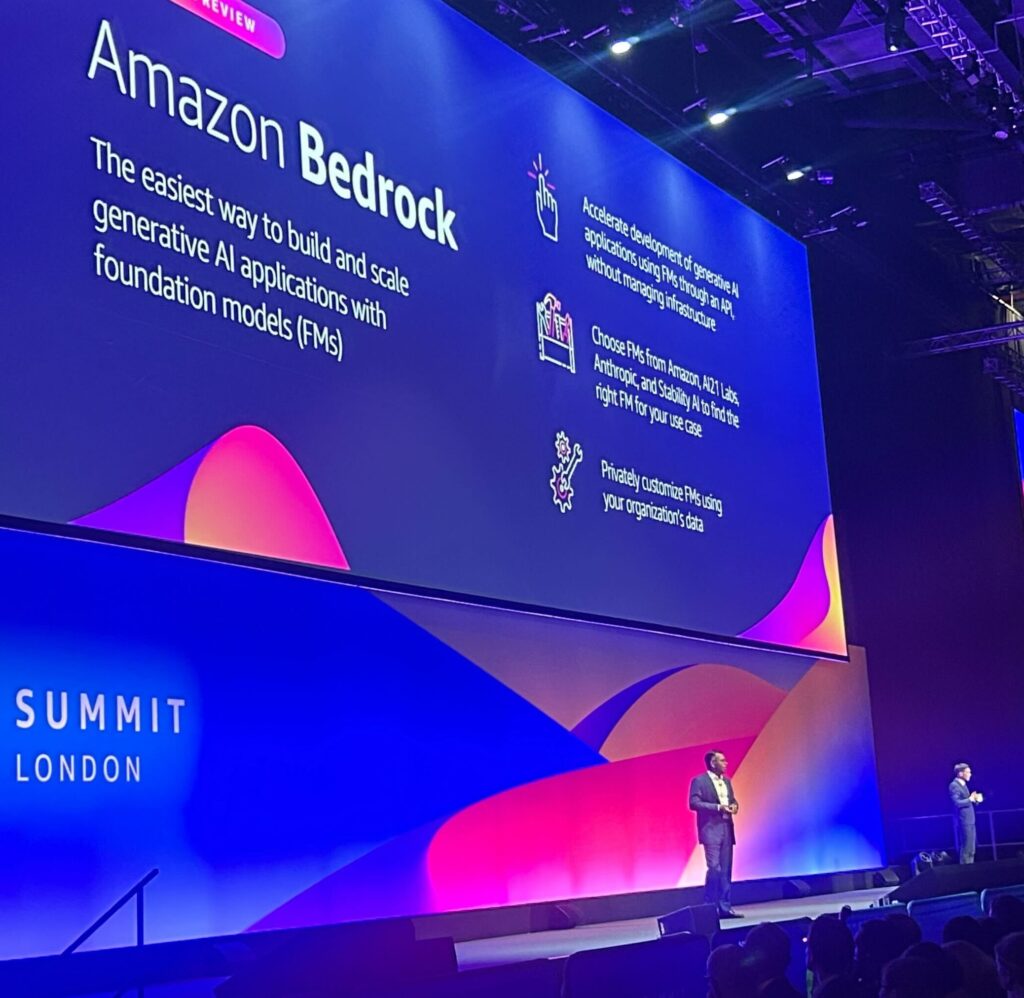
AWS has been a vocal proponent of “democratizing” AI in recent months, partnering with data and machine learning platform Hugging Face to provide trusted open models. Sivasubramanian rejected the idea that any one model could meet the use cases of every customer all the time, in contrast to competitors like Microsoft, which have focused on powerful all-rounder LLMs such as GPT-4.
Different models vary in their effectiveness when handling tasks such as non-English languages or tone of voice, Sivasubramanian explained. He also acknowledged that businesses currently lack concrete measures to benchmark AI models for specific use cases. However, he suggested that companies would eventually learn which models are best suited for various tasks through exposure over time.
AWS’s API-led approach allows firms to freely explore which models are best suited for their purposes on a trial-and-error basis. This flexibility could prove especially useful for smaller firms, which may not have the expansive metadata necessary to tailor a model to fit their specific company brand to a significant degree.
By focusing on a diverse AI ecosystem that caters to specific applications and use cases, AWS is distinguishing itself from competitors and forging its own path in the world of generative AI.